The Magic School Bus is the pinnacle of children’s television. Optimistic, fanciful, and lighthearted, the show does what entertainment should do: engage a curious audience. In one of my favorite episodes, “Inside Ralphie,” Ms. Frizzle and the gang shrink down and venture inside their sick classmate’s body. Evading bacteria and white blood cells, the class narrowly escapes and is eventually sneezed out. Although entertaining, ruminating on how Ms. Frizzle and the class explored Ralphie is pointless; it’s a kid’s show and shrinking technology doesn’t exist. Vehicles that navigate the human body, however, do.
Small molecular particles made of fatty bilayers, known as lipid nanoparticles (*LNPs), have emerged as a promising drug delivery vehicle due to their stability, biocompatibility, low toxicity, and sustained cargo release. LNPs are famous for their ability to encapsulate DNA, mRNA, and/or protein-based therapeutics, and carry them to specific target sites within the body. Used to deliver Pfizer and Moderna’s COVID-19 vaccines, several LNP drugs across different indications are currently FDA-approved and/or in clinical trials.
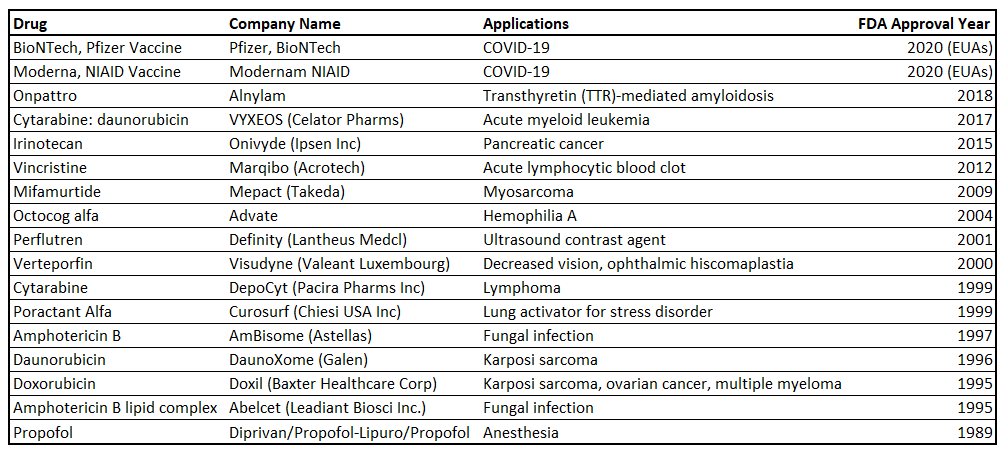
Current issues with targeting, cytotoxicity, complex manufacturing, and solubility remain. As a result, researchers have turned to biologically native particles known as extracellular vesicles (EVs) for inspiration due to their origin and lipid-bound membranes. Although a limited amount of studies exist, the number of papers experimenting on EVs as drug-delivery vehicles has accelerated in recent years. Current studies focus on improving blood circulation time, intrinsic targeting, cellular uptake, and reducing cytotoxicity, i.e., optimizing vesicle structure. These structural optimizations and iterations on development approach techniques are, in my view, the key considerations for future growth and expanded use cases for lipid nanoparticles.
Composition and Optimization
When administered intravenously, LNPs are at the will of the reticuloendothelial/mononuclear phagocyte systems (RES/MPS). Unmodified vesicles are often captured and deposited in the liver, spleen, and lungs before arriving at their targeted tissues/organs. To address this, iterations on vesicle composition focus on changes to membrane formulation, lipid charge, lamellarity, sizing, head and tail group characteristics, mechanics, and fluidity modulation.
Composition
The composition of LNP and EV membranes varies based on the objective. How potent is the cargo? How long does it need to last in circulation? What’s the target organ/tissue? What lipid types are used? How is the membrane packed? LNPs are made of four lipid groups: an ionizable lipid, a phospholipid, cholesterol, and a PEGylated lipid. Ionizable lipids are typically synthetic whereas others are natural. Differences in lipid headgroup, chain length, chain saturation, and packing impact how cells recognize vesicles in the body and how effectively they’re taken up by tissues. Cholesterol, for example, enhances the stability of LNPs in circulation but also influences their uptake in RES-rich organs.1
On the membrane, lipid headgroups influence a vesicle’s net charge, affecting both uptake and clearance rates. Cationic lipids will induce a positive charge on the membrane surface and negatively charged ones the opposite. EVs will typically carry a net negative charge whereas, for LNPs, the constraint is more regulated. For nucleic acid therapies, positively charged LNPs are typically used at a low pH (which enables RNA complexation) and neutral at physiological pH (which in turn reduces toxic effects).2
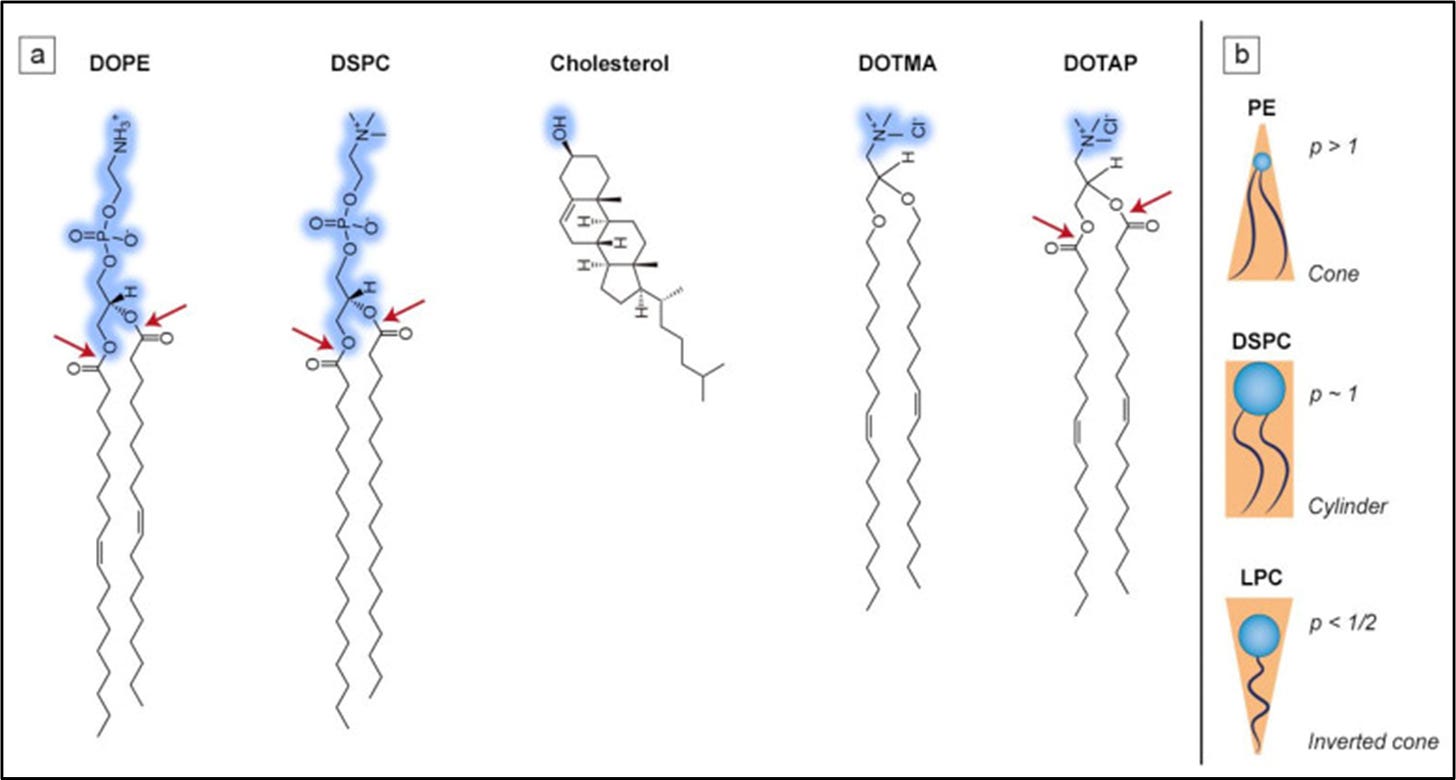
Another part of vesicle composition is lamellarity or the presence of internal bilayers in the nanoparticle. LNPs are categorized as unilamellar (a single outer lipid bilayer), multilamellar (consecutive, concentric lipid bilayers in a single outer lipid bilayer), or multivesicular (separate, smaller-sized vesicles contained within a single outer lipid bilayer). The number of internal membranes influences how a payload is encapsulated and its release timing.
Size is also an important composition component because it establishes the carrying capacity of the vesicle itself in addition to cellular uptake. Ranging from 10nm to 1000nm, nanoparticles are classified as small, large, and giant. Sizing depends on the formulation process used to create the nanoparticle. Because of their size, LNPs have high curvatures and appropriate membrane packing is essential to maintain their stability. Cholesterol is often relied upon to fill in membrane gaps and hold the nanoparticle together. Outside of experimenting with composition, other approaches to improve LNP performance include PEGylation, membrane proteins, and small molecule integration.
Optimization
PEGylation, or coating LNPs with polyethylene (PEG), is a common optimization approach. PEG shields liposomes from interactions with plasma proteins, therefore, improving circulation time in vivo. Naturally hydrophilic, PEGylated lipids protect LNPs from aggregation, opsonization, and uptake by white blood cells. The strength of PEGylation depends on the lipids’ chain length and density. Both Moderna and Pfizer used PEGylated lipids in their COVID-19 vaccines (DMG-PEG 2000 and ALC-0159).3
LNPs are traditionally made up of only lipids. EVs, on the other hand, naturally have membrane proteins. Recently, researchers have begun including proteins in LNP membranes at the influence of EVs. Dysoponic proteins like CD47 are used to evade phagocytic clearance. Antibodies, targeting peptides, and antibody fragments are used to increase intrinsic targeting. Virus-inspired peptides, such as GALA-peptide, albumin, gap-junction proteins like CxC43, and fusogenic peptides are used to enhance cellular uptake and cytoplasmic delivery.456
Outside of PEGylation and protein integration, other attempts at LNP optimization include incorporating small molecules such as Glutathione and folic acid in the surface membrane.7 DNA, RNA, and pH-responsive aptamers are also used for enhanced targeting capabilities in vivo.8 One of the more interesting optimization approaches is integrating iron-based nanoparticle modifiers into LNPs for magnetic responsiveness. These iron-based modifiers are controlled by an external magnetic field for targeting.9
Overall, experimentation on LNP Structure largely revolves around their composition, PEGylation, membrane protein integration, and small molecule integration.
Future Approaches
LNP/EVs as a drug delivery system are still early. As more papers are published, the number of approaches augmenting and optimizing LNP/EVs only accelerates. Two areas that I’m following are 1) combined approaches, and 2) computational approaches
Combined Approaches
Research on EVs as a drug delivery system is limited. As previously mentioned, scientists have begun to use EVs as a source of inspiration, i.e., incorporating their mechanisms and design into LNPs. These combined approaches are known as hybrid vesicles and EV-inspired liposomes. Hybrid vesicles are EVs that incorporate liposomal components tuned for specific traits such as enhanced stability, circulation time, or targeting properties. On the other hand, EV-inspired liposomes are LNPs that include the composition characteristics of natural vesicles. EV-inspired liposomes retain the control of traditional synthetic LNP design but incorporate naturally occurring proteins and lipids found on EV membranes. Both approaches enhance the desired components/traits that support delivery efficiency, stability, circulation time, and targeting properties.10 As of now, combined approaches have been tested to deliver siRNA-based therapeutics in comparison to both traditional LNPs and EVs. With this said, a large amount is still unknown about how EV composition affects functionality and performance. Until more research is done, combined approaches remain a hope rather than a reality.
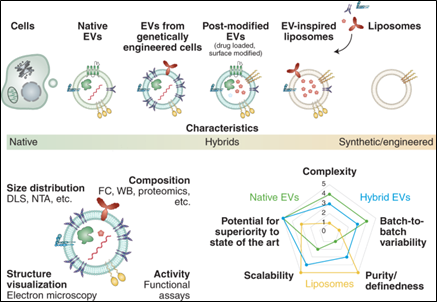
Computational Approaches
Computer-aided drug development companies, now referred to as ‘techbio,’ have captured headlines for the past decade. Names like Recursion, BenevelentAI, and AbCellera, amongst others, have raised hundreds of millions of dollars to apply machine learning, computer vision, and natural language processing techniques to biological data to bring candidates to market faster. As these companies build different targeting strategies and development platforms, it only makes sense that these techniques are applied to LNPs.
Current attempts at computational approaches deliberate on connecting experimentally measured properties and molecular mechanisms of LNPs. Although several modeling approaches exist, most research focuses on molecular dynamics (MD) simulations targeting lipid bilayers, PEGylated membranes, and larger liposome simulations.
Lipid Bilayer Simulations
As previously mentioned, membrane formulation is the most important factor in LNP performance and optimization. To best design LNPs, an understanding of how permeable membranes is needed. Traditional experiments are poor at understanding the dynamic diffusion process and often can’t separate diffusion and partitioning in a permeability study. MD simulations, have proven to be effective at this, and studies looking at permeability across lipid bilayer sections are often used to infer results.1112
PEGylated Membrane Simulations
Simulations on PEGylated nanoparticles have also been studied in an attempt to understand 1) how PEGylation improves membranes’ drug loading efficiencies, and 2) how PEG interacts with LNP components, e.g., cholesterol. For drug loading efficiency, one recent experiment looked at how hydrophobic molecule 5,10,15,20-tetrakis (4-hydroxyphenyl) porphyrin (p-THPP) interacts with lipid bilayers. This study ascertained that p-THPP had greater solvent exposure in PEGylated liposomes than in zwitterionic ones.13 Looking at how PEG interacts with other LNP components, researchers have shown that PEG enters the lipid bilayer by aligning with the β face of the cholesterol molecule. Moreover, PEG interferes with cholesterol’s role in structuring and compacting the membrane, i.e., when the LNP membrane is PEGylated, the area per lipid increases with increasing cholesterol.14
Liposomal Simulations
Because MD simulations operate at a small scale (0.1nm-100nm), larger-scale structures and LNP dynamics aren’t often reproducible. Subsequently, researchers have attempted to use coarse-graining molecular dynamic simulations (CGMD) to observe them. This approach preserves most structural properties of individual molecules in liposomes while providing the opportunity to investigate biological dynamics.
A popular model for liposomal simulation is the MARTINI CG model. The MARTINI CG model is consistently a favored choice due to its ability to assess physical stability. Studies using this model have observed that closely packed lipid heads and disordered lipid tails are found in LNP inner monolayers while the opposite was seen in the outer monolayer.15 Additionally, CGMD simulations have been used to evaluate the performance of liposomes as drug carriers. One study looked at hypericin, a photosensitizer for photodynamic therapy, and found close to the polar headgroup of a liposome’s outer lipid layer to maximize interactions using its more hydrophobic end with the lipid interior.16
Companies & Constraints
As of now, techbio companies using computational approaches for vesicle design don’t exist at scale; A few startups, are trying, however. Namely Mana.Bio, Sana Biotechnology, and ReCode Therapeutics for LNPs and Codiak, Evox Therapeutics, STRM.Bio, and SYENEX for EVs. These companies are focused on optimizing vesicle-based delivery systems early and proof of concept has yet to be shown. On top of these startups, Acuitas has operated in the LNP space for the better part of the last ten years. Using a rational design approach to LNP creation, Acuitas has synthesized over 500 novel lipids and brought the polyneuropathy drug, Onpattro, to market in 2018 in conjunction with Alnylam. Although clinically proven, the exact computational approaches used by Acuitas are not public.
As the number of computational experiments, approaches, and companies centered around LNP design and development grow, a problem that currently exists within this space is establishing relevant quantitative structure-activity relationship (QSAR) models linking LNP behavior between in vitro and in vivo. An increasing amount of time and money has been invested in searching for a formula between composition and clinical behavior. Although a few studies successfully predict the phase behavior of lipid formulations with different ratios of fatty acids, antibacterial profiles, and toxicity, accurate and reliable simulations are not guaranteed17
Thoughts
LNP/EVs are a promising option for drug delivery vehicles. With new LNP drugs entering clinical trials each year, the opportunity for novel formulations and computationally focused development platforms is massive. With this said, building a development platform is time-consuming and highly dependent on the utilized lipid library.
I wonder why computationally focused platforms don’t already exist on a larger scale. It could be due to a lack of combinatorial data available or a lack of resources. My hunch is timing and an overly competitive lipid patent landscape. LNP/EV drug delivery isn’t preferred (or potentially possible) for most therapeutics yet. Today, there are fewer FDA-approved oligonucleotide therapies compared to small molecules and/or biologics. As a result, market demand isn’t strong enough that a leading computational platform has developed. On top of this, more LNP drugs enter trials, the patent landscape is becoming increasingly congested, and what’s considered infringement requirements remain unknown. As of August 2022, several lawsuits have been filed by Acuitas and Alnylam against Pfizer, BioNTech, and Moderna over lipid design and formulation. Although there haven’t been any outcomes yet, the decisions on these lawsuits will likely influence the approach that companies take for LNP development moving forward.
With the number of marketed LNP drugs, candidates in clinical trials, and the coming wave of oligo-based therapies, the need for novel non-viral delivery systems escalates. Current approaches to LNP/EV improvement focus on vesicle composition and integrating PEGylation, proteins, and other small molecules into the membrane. I think the winners in this space will be companies that develop platforms that leverage unique lipid libraries and can treat different disease types. Easier said than done, the future of LNP/EVs will be the future of therapeutic delivery; something that I’m sure Ralphie and Ms. Frizzle would appreciate.
*Although there’s different classifications for what exactly constitutes a lipid nanoparticle, for the sake of simplicity, LNPs will be used in reference to all lipid-based nanoparticles in this post.
Thank you to Denis, Matt S., and Matt D. for thoughts and feedback. Opinions are my own and do not reflect the views of any affiliate organizations.
Very insightful, well written and timely article!!